EpyDiagnosis: The New Era of Medical Diagnosis
EpyDiagnosis is a pioneering platform, redefining early lung cancer detection with a novel approach that extends beyond a simple model enhancement
- Custom Dataset: Crafted from real patient data in Mexico, our tailored dataset underpins a solution deeply attuned to the local demographic, ensuring relevance and accuracy.
- Image Processing: We’ve automated the conversion of DICOM files to image data, incorporating advanced normalization and cleaning techniques to prime the data for AI analysis.
- Model Innovation: Beyond modifying EfficientNetB0, we’ve built an integrated system that encapsulates data curation, model training, and deployment within a single streamlined pipeline.
- API Deployment: Our model is accessible through an API designed for ease of integration, offering versatility across various healthcare applications and systems.
Dataset Origin
The dataset under analysis was sourced from the IMSS database, covering the period from January to June 2023, with a total of 720 documented cases.
Data Classification and Preparation
Through a detailed process of data classification and curation, we compiled a consistent set consisting of 3,800 malignant cases, 5,800 normal cases, and 520 benign cases. We adhered to the standard data split distribution of 70/20/10 for training, validation, and testing, respectively, to ensure model generalization.
Model and Architecture
We deployed a modified version of the EfficientNetB0 model, tailored to the specific characteristics of the medical images acquired, to optimize the detection of relevant patterns in the computed tomography data.
Performance Evaluation
The final evaluation of the model yielded a loss of 0.0508 and an accuracy of 98.17%. These results indicate an outstanding performance in the classification of the test images.
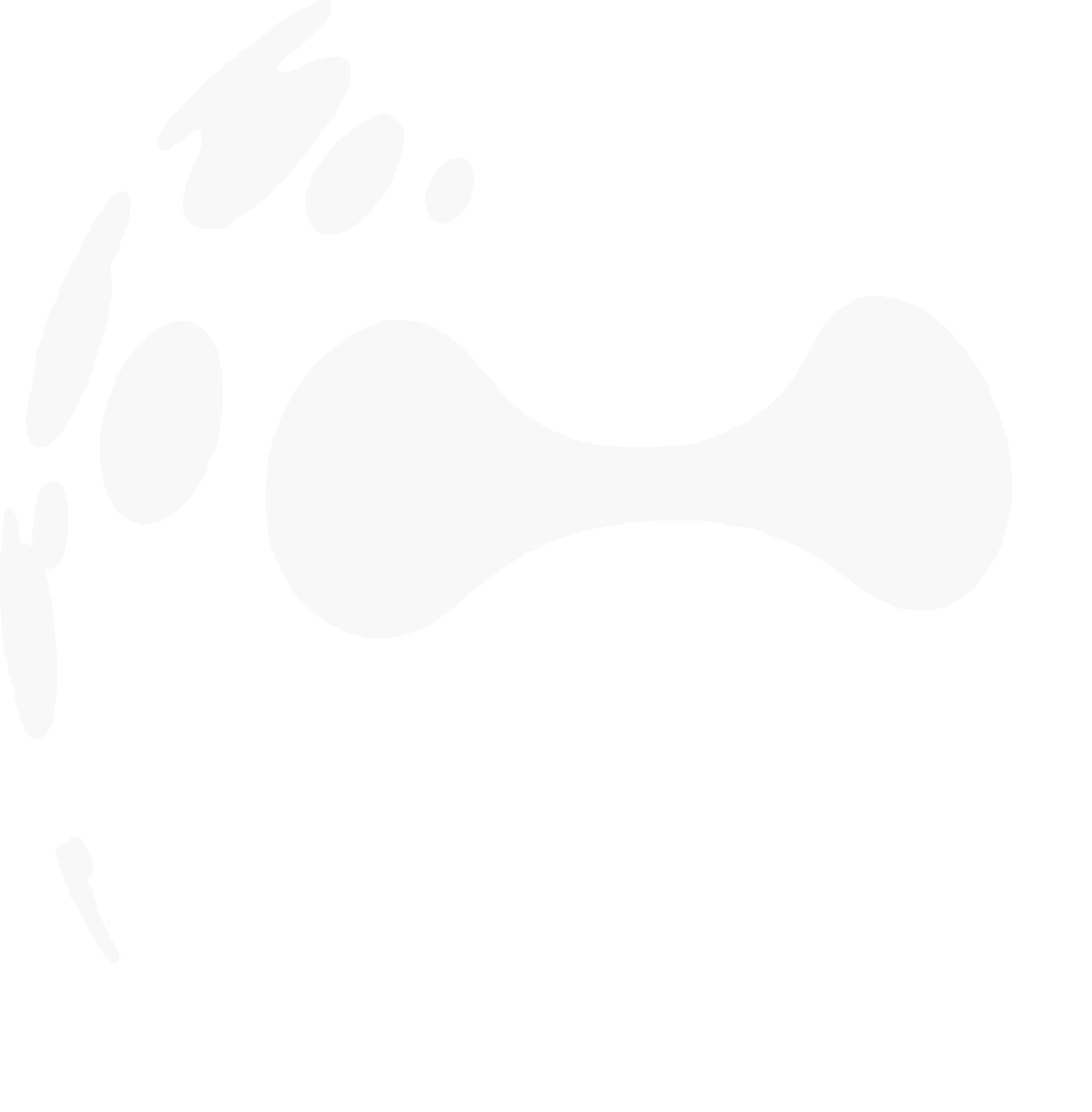
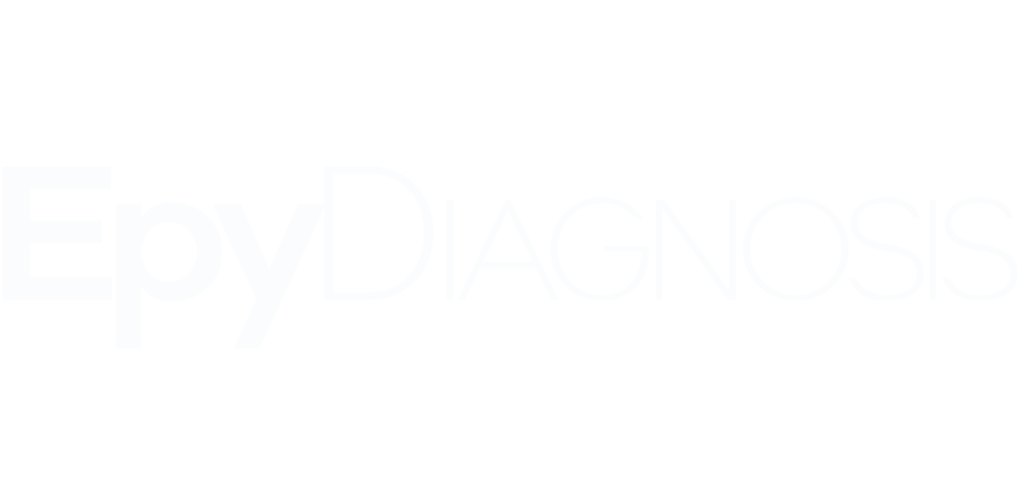
Training and Validation Metrics Analysis
The accuracy and loss graphs show peaks suggesting overfitting at certain stages of training, indicating that the model might be too specialized in the training set and thus, may not generalize well to new data.
Improvement Strategies and Future Steps
In response to the observed overfitting peaks, EpyDiagnosis commits to undertaking the following actions
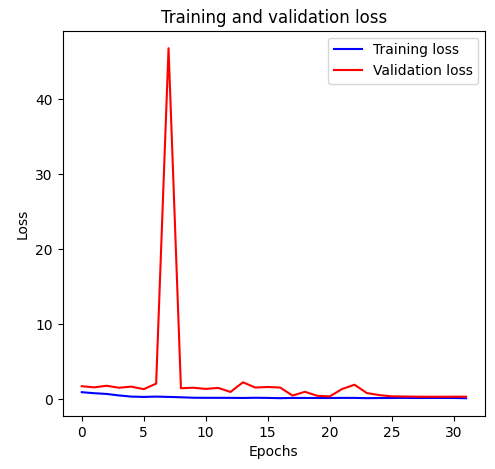
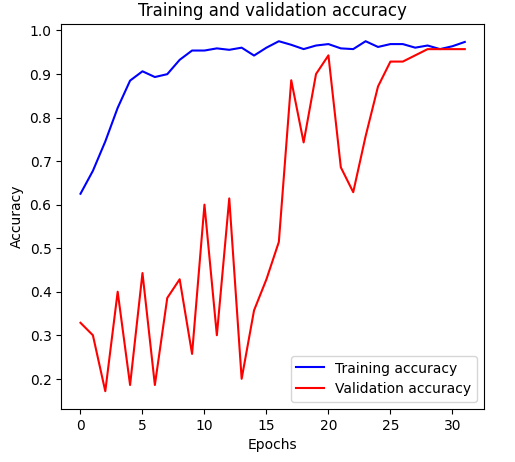
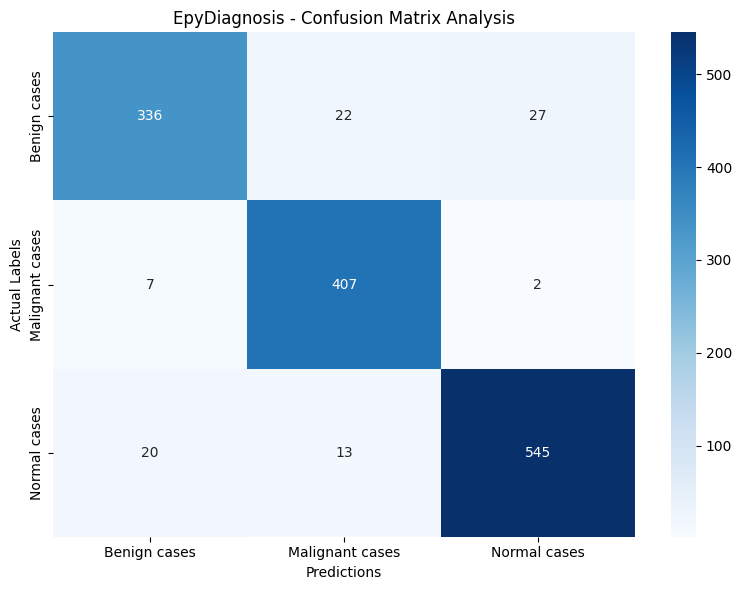
- Development of a New Model: We will construct a second model, applying additional regularization techniques and fine-tuning to mitigate overfitting and enhance the model’s generalization ability.
- Dataset Expansion: We will incorporate additional data corresponding to the period from January to December 2023, to enrich the training set and improve the model’s robustness against a wider variety of cases.
- Continuous Validation: We will perform iterative validation of the new model, using a broader and more diverse sample of images to ensure consistency and reliability of the predictions.
What Sets Us Apart
Domain-Specific Adaptation: We’ve customized EfficientNetB0 to cater to the unique features of pulmonary computed tomography (CT) images. This includes adjustments in the model’s architecture and image preprocessing algorithms to enhance the detection and classification of lung nodules in a real clinical context.
Focus on Data Quality: Data quality and representativeness are crucial for training any AI model. At EpyDiagnosis, we meticulously select and curate our datasets from IMSS, ensuring our model is trained on data that is highly relevant and specific to the population we aim to serve.
Robustness and Generalization: By expanding our dataset to include a greater variety and implementing techniques to prevent overfitting, our model becomes more robust and can generalize better to new cases than standard models, which is vital for reliable performance in clinical settings.
Integration and Accessibility: The EpyDiagnosis model is designed for seamless integration with hospital IT systems via an API, facilitating its adoption and use in daily medical practice. This means it can be inserted into the existing workflow with minimal impact on current hospital operations.
Response to Local Clinical Challenges: Our tool is developed with a deep understanding of the specific challenges faced in the Mexican healthcare system, making it particularly suited to meet these unique needs, unlike generic solutions that may not be as effective in this context.
Commitment to Affordability: EpyDiagnosis is committed to keeping our solution affordable and accessible, especially for developing markets where the burden of lung cancer is high and resources are often limited.
Continuous Improvement: We are dedicated to continuous improvement based on clinical feedback and technological advancements, ensuring our model remains at the forefront of AI technology in medicine.